SummAct: Uncovering User Intentions Through Interactive Behaviour Summarisation
Guanhua Zhang, Mohamed Ahmed, Zhiming Hu, Andreas Bulling
Proc. ACM CHI Conference on Human Factors in Computing Systems (CHI), pp. 1–17, 2025.
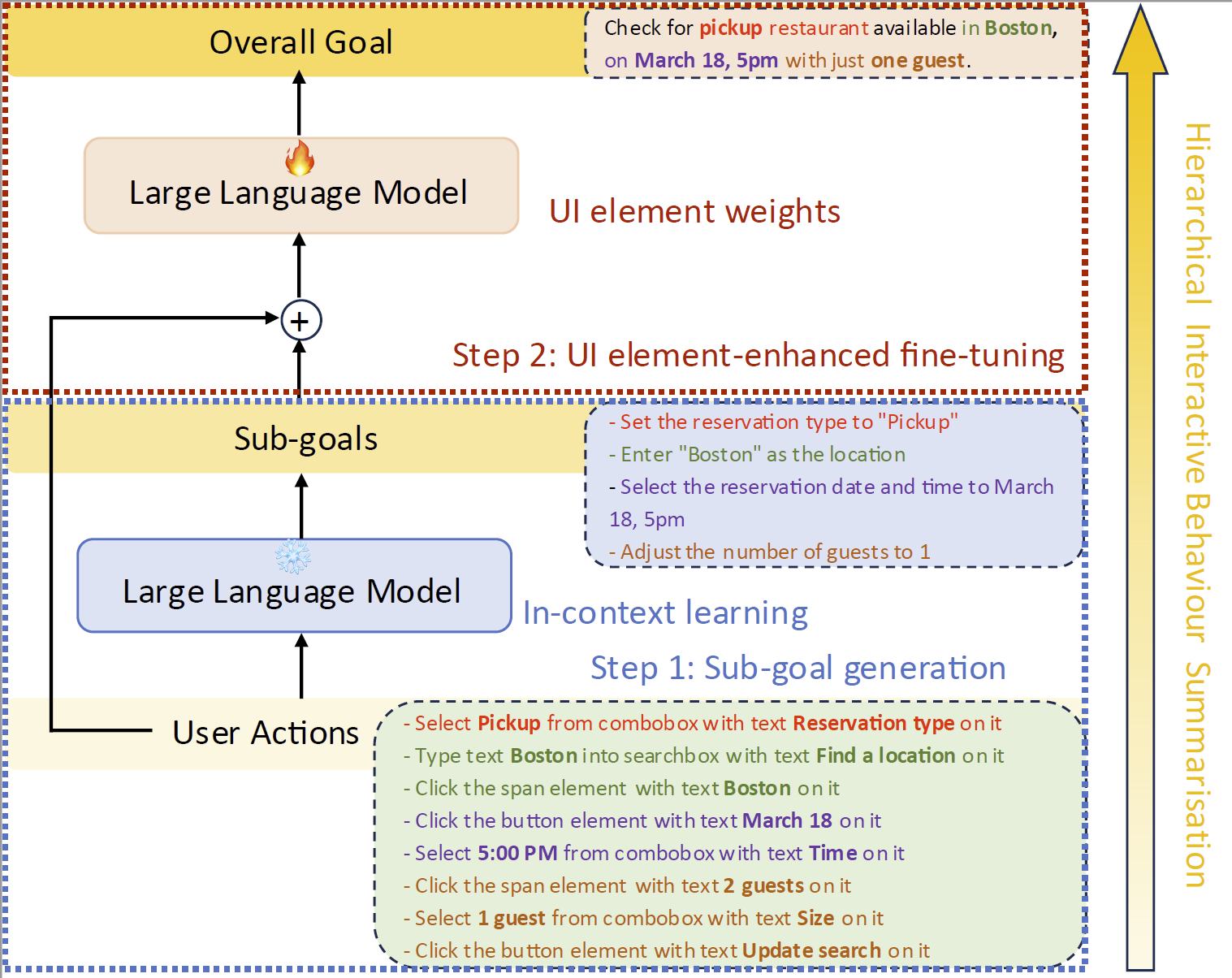
Abstract
Recent work has highlighted the potential of modelling interactive behaviour analogously to natural language. We propose interactive behaviour summarisation as a novel computational task and demonstrate its usefulness for automatically uncovering latent user goals while interacting with graphical user interfaces. We introduce SummAct – a novel hierarchical method to summarise low-level input actions into high-level goals to tackle this task. SummAct first identifies sub-goals from user actions using a large language model and in-context learning. In a second step, high-level goals are obtained by fine-tuning the model using a novel UI element weighting mechanism to preserve detailed context information embedded within UI elements during summarisation. Through a series of evaluations, we demonstrate that SummAct significantly outperforms baseline methods across desktop and mobile user interfaces and interactive tasks by up to 21.9%. We further introduce two exciting example use cases enabled by our method: interactive behaviour forecasting and automatic behaviour synonym identification.Links
BibTeX
@inproceedings{zhang25summact,
title = {SummAct: Uncovering User Intentions Through Interactive Behaviour Summarisation},
author = {Zhang, Guanhua and Ahmed, Mohamed and Hu, Zhiming and Bulling, Andreas},
year = {2025},
pages = {1--17},
booktitle = {Proc. ACM CHI Conference on Human Factors in Computing Systems (CHI)}}